“We must be bold enough to embrace all the potential with open eyes because one thing is certain: the next new idea is just around the corner and it might just save a life.” – Arif Husain, WFP
Data innovations hold promise for addressing challenges across all aspects of the nutrition data value chain (DVC). The time is opportune to leverage new data sources, methods, and technology due to the increased need for high quality and real-time data brought about by the COVID-19 pandemic. Recent convenings such as the UN World Data Forum in October 2021 have also featured data innovations as a key theme to showcase the potential of new solutions.
Given the limited work around data innovations for nutrition, DataDENT conducted a landscape analysis of trends and opportunities for nutrition data innovations. We reviewed data innovations developed in or after 2015 across the following nutrition domains—diet, food security, food environment, food fortification, micronutrients, and nutrition status.
Data innovations are the use of new or non-traditional data sources, methods, and partnerships to reframe issues and generate new solutions to existing nutrition data challenges.
To guide our review, we first identified data innovation categories and their sub-categories which we found to be most prominent in the broader data innovation space (Figure 1). We then mapped the 60+ nutrition data innovations found in our search to these categories. We found a significant number of nutrition data innovations are mobile solutions, digitalization, or artificial intelligence. We also found the majority of innovations to be related to nutrition status and diet data, with comparatively less around food fortification data. Finally, most innovations influence the collection, analysis, and translation of data and less so which data to collect.
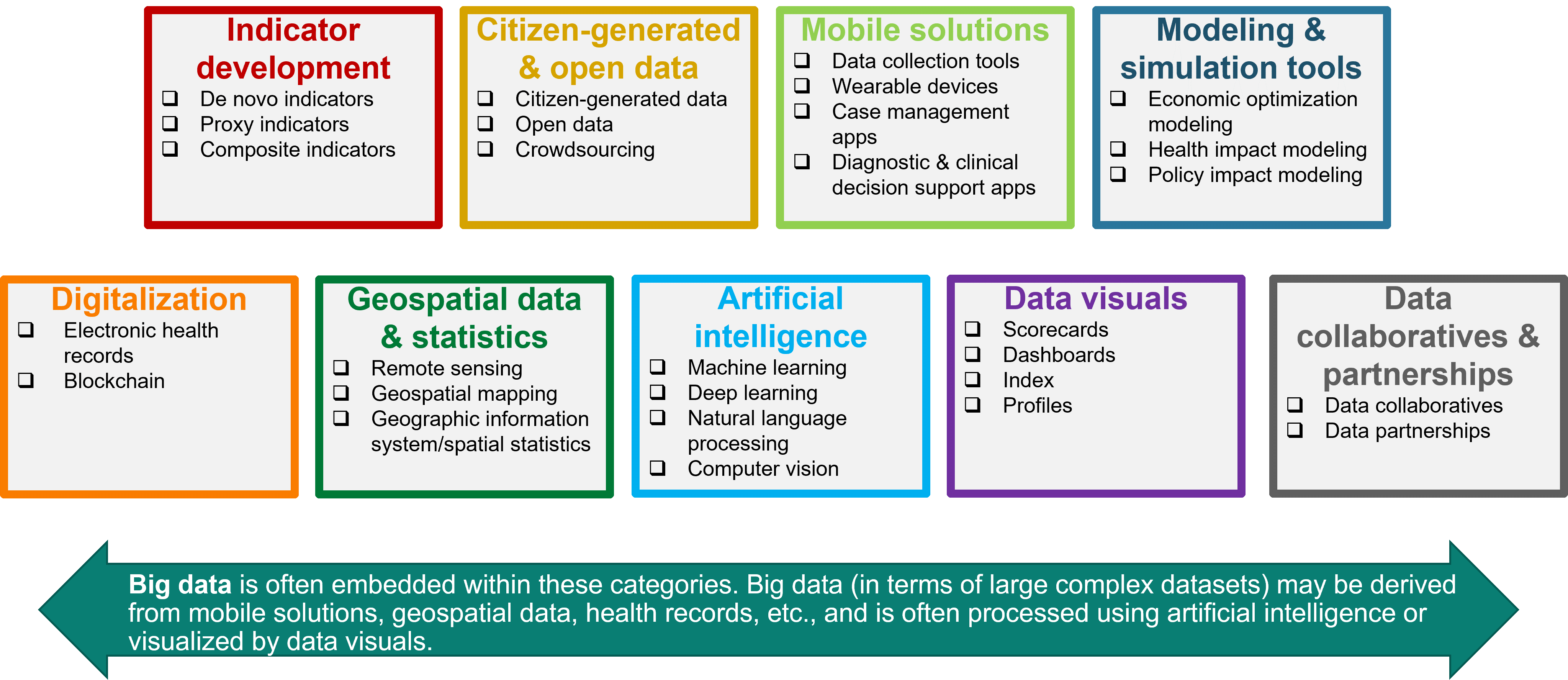
Figure 1: Data Innovation Categories and Sub-categories
1. Mobile Solutions
Mobile solutions are used to increase the efficiency, ease, and quality of data collection, storage, and analysis (often when paired with other innovations on the backend). Some mobile apps enable remote data collection in hard-to-reach populations or during emergencies like the COVID-19 pandemic where in-person data collection is not feasible (e.g., Mobile Vulnerability and Analysis). Others aide in case management (e.g., CommCare for Nutrition) as well as diagnostic and clinical support, frequently using intelligent algorithms and artificial intelligence (e.g., Child Growth Monitor). However, it is important to note mobile platforms may exclude people who do not have phones and some mobile applications require connection to Wi-Fi which may not always be available or stable.
Sanku-Project Healthy Children created a dosifier technology to enable small flour mills in East Africa to fortify flour with the precise amount of nutrients. This technology was equipped with cellular connectivity which grants access to real-time data on maintenance, machine tracking, nutrient contents, and performance via GPS and automatic curation into a central, cloud-based dashboard. This solution transforms the data collection process by allowing mills to be remotely monitored, optimizing performance and ensuring high quality of the fortified food products.
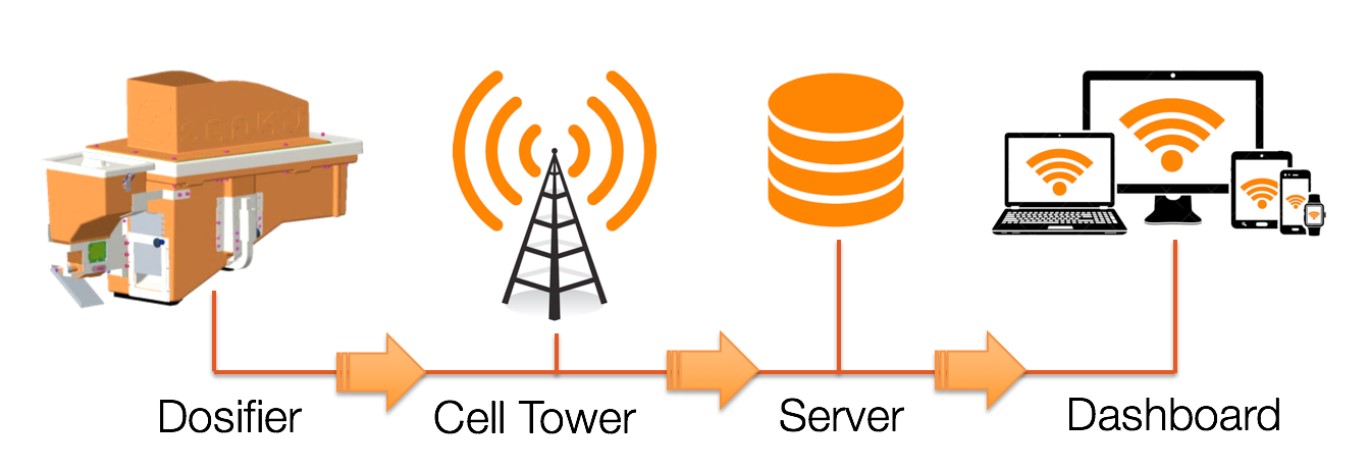
Figure 2: Sanku Dosifier data collection process
2. Artificial Intelligence
Artificial intelligence (AI) is a booming field of data innovation across many sectors that allows computer systems to perform tasks which normally require or are beyond human intelligence such as visual perception, speech recognition, language processing and decision-making. Within nutrition, we found artificial intelligence to be mainly used for predictive analysis and forecasting for food and nutrition security (e.g. Nutrition Early Warning System) as well as to provide diagnostic and decision support around nutrition status (e.g., Count Me In and SAM Photo Diagnosis App). While AI does bring new capabilities to the data space, it is not always feasible given its implementation requires specialized technical knowledge and equipment which can store and process large amounts of data.
The HungerMAP Live was created by the World Food Programme to provide near real-time monitoring of food security in more than 90 countries in a visual format. The system uses artificial intelligence to power predictive models which estimate acute food insufficiency by pulling together and analyzing data from a variety of different sources including weather, population size, conflicts, nutrition, and food security. AI is also used to produce estimates of food security where data is missing to present a comprehensive depiction of the situation.
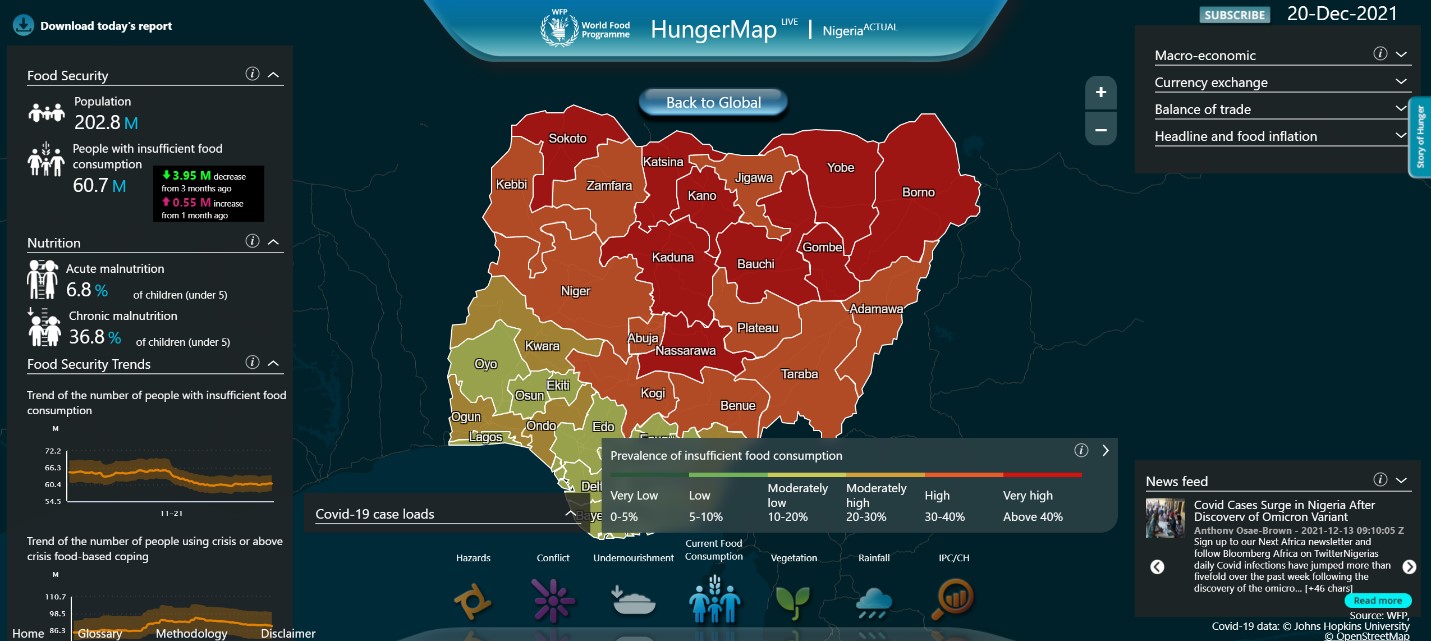
Figure 3: Screenshot of the HungerMAP Live in Nigeria
3. Citizen Generated Data
Citizen generated data (CGD) is the method of sourcing data directly from citizens. CGD brings a new perspective compared to traditional data collection methods by allowing community members to directly contribute their personal experiences and opinions, especially those who are commonly missed or excluded in traditional efforts. However, it is important to ensure the data complies with appropriate quality standards given it is often collected through unstructured interviews/discussions, mobile phones, or online platforms (e.g., Twitter). Within nutrition, this method can be used to generate data on the perception, attitudes, values, and norms around a variety of topics such as food consumption (e.g. Citizen H2D3), food access (e.g., Food Price Crowdsourcing Africa), and nutrition service utilization.
Citizen Voice and Action is a social accountability approach developed by World Vision which facilitates communication between communities and government to identify service gaps and advocate for solutions. This unique community level data collection method has been successfully used in Kenya to increase the number of children screened and treated for malnutrition based on data from citizens advocating for improved service delivery and additional health workers. The Citizen Voice and Action approach is innovative because it gathers critical information from community members who utilize nutrition services directly and therefore can provide a more accurate depiction of the situation.
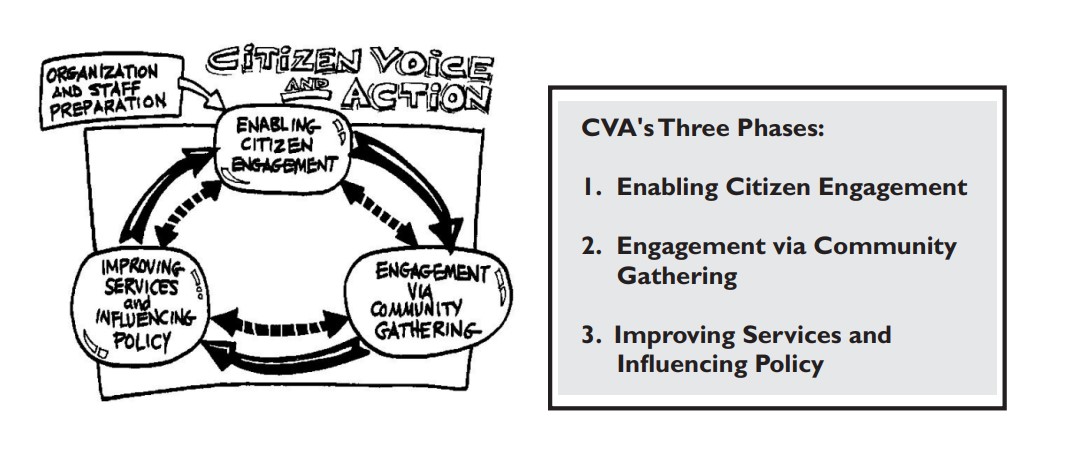
Figure 4: Three Phases of Citizen Voice and Action
Data innovations present opportunities for nutrition, whether by adopting, adapting and scaling existing nutrition data innovations—or adapting data innovations used in other sectors for nutrition. If you are interested in learning more about how other data innovation categories play a role in nutrition data, we encourage you to read more on the DataDENT website here.
Acknowledgements
The authors gratefully acknowledge comments on this blog post from Augustin Flory (Results for Development Senior Fellow) and Rebecca Heidkamp (Johns Hopkins Bloomberg School of Public Health).